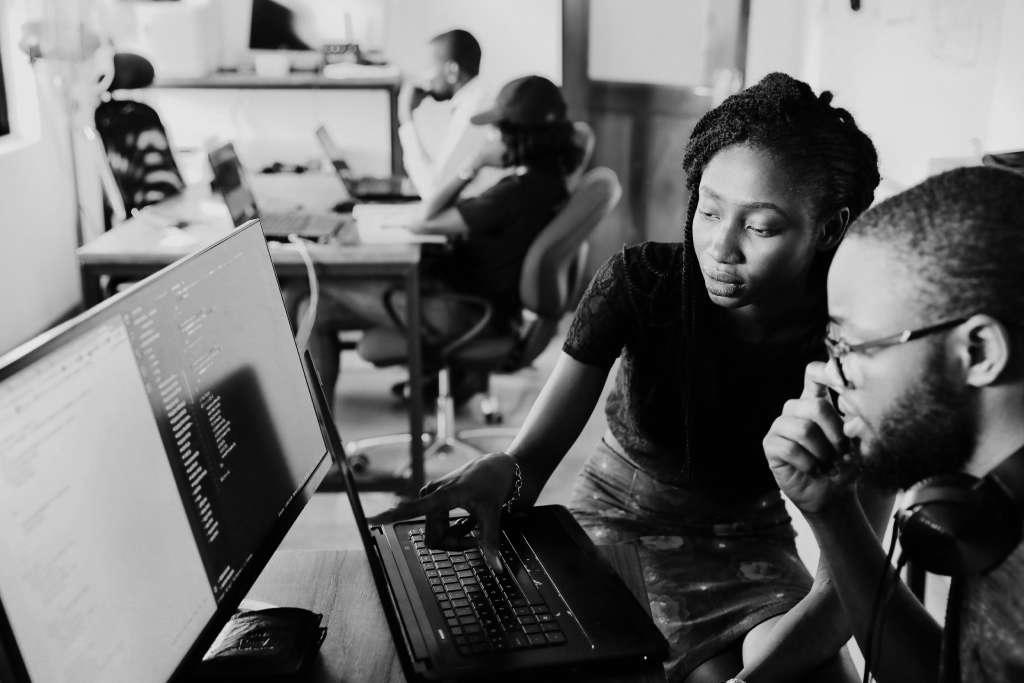
Secure analytics platform for NHS electronic health records
OpenSAFELY delivers research across over 58 million people's health records, always respecting patient confidentiality
Better research, improved patient confidentiality
Why OpenSAFELY?OpenSAFELY is a highly secure, transparent, open-source software platform for analysis of electronic health records data. All platform activity is publicly logged. All software for data management and analysis is shared, automatically and openly, for scientific review and efficient re-use.
Protecting privacy
We never let researchers download patient data, and OpenSAFELY tools let users to write code to analyse patient data without even needing to view the raw records.
Auditable by the public
It is a privilege to use patient data for the public good. OpenSAFELY respects patients by carefully considering this in every part of its design.
Better, open science
OpenSAFELY requires publication of all analytic code, and our tools drive all users to produce prespecified, reusable, testable, shareable and modular software for research.
Enabling high volumes of research
Over 60 published research outputs to date, with many more in progress.
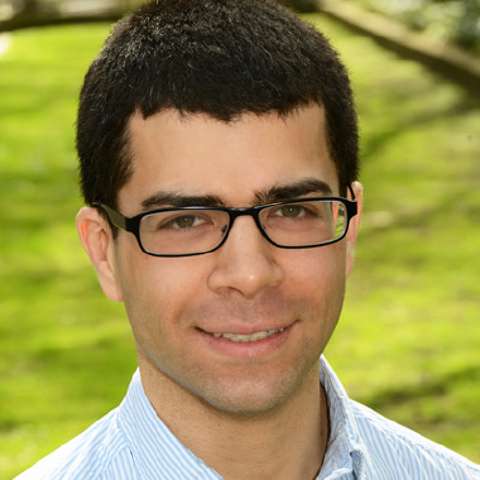
OpenSAFELY is revolutionising the way we work with health data. The initiative has already delivered major contributions to public health during the COVID-19 pandemic. But OpenSAFELY also leads the way in terms of transparency, open working methods, and a uniquely secure data access model.
Ready to learn more? Get started within an hour
Open for research
We are currently working with NHS England to cautiously on-board a small number of external pilot users to develop their analyses on OpenSAFELY. This first wave of pilot users will be collaborators, working closely alongside us to co-develop the platform.
Read about our pilot onboarding processPython, R and Stata
Choose any of these languages to write your analytic code
Primary care data
Our deployment for NHS England uses primary care data to research questions related to the Covid-19 emergency
A commitment to open ways of working
We believe transparency and open working methods are key to earning public trust and improving research quality
Latest from the blog
Join us for our 2nd Annual OpenSAFELY Community Symposium!
Two days of talks and workshops on research, data infrastructure, and open science.
Recording technical decisions using ADRs
The Research Experience team explain how they’ve been recording decisions
We won an MRC award for open science
Most of the time, we like to keep things fairly formal on this blog - but if you’ll forgive us a small indulgence, today we’re going to blow our own trumpet a little. A couple of weeks ago, my colleague Rose Higgins and I went to an event in London where this Institute was awarded the Open Science Impact award, as part of the MRC Impact Prize. We were, needless to say, over the moon about it.
Research-ready computers in the cloud
Spend more time answering your research questions – and less time wrangling your computer – with GitHub Codespaces and the OpenSAFELY development container.
How COVID-19 affected sickness absences in England
Our new study looks at the rate that sick notes were issued for people with COVID-19, and how it changed over the course of the pandemic.
No increase to opioid prescribing during the COVID-19 pandemic in England
During the COVID-19 pandemic, there were no sustained increases in opioid prescribing.